SEZ-HARN: Self-Explainable Zero-shot Human Activity Recognition Network
| #Deep Learning #Zero-shot Learning #Explainable AI #Temporal Modeling #Pytorch
Abstract
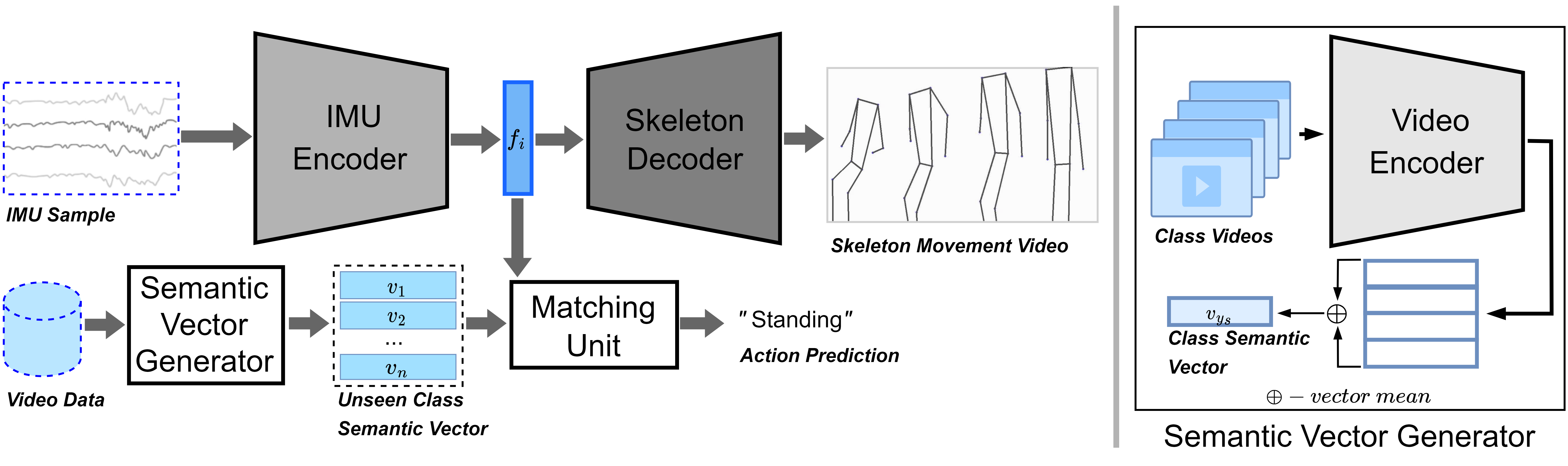
Human Activity Recognition (HAR) using data from Inertial Measurement Unit (IMU) sensors has many practical applications in healthcare and assisted living environments. However, its use in real-world scenarios has been limited by the lack of comprehensive IMU-based HAR datasets that cover a wide range of activities and the lack of transparency in existing models. Zero-shot HAR (ZS-HAR) overcomes the data limitations, but current models struggle to explain their decisions, making them less transparent. This paper introduces a novel IMU-based ZS-HAR model called the Self-Explainable Zero-shot Human Activity Recognition Network (SEZ-HARN). It can recognize activities not encountered during training and provide skeleton videos to explain its decision-making process. Experiment results on four benchmark datasets (PAMAP2, DaLiAc, UTD-MHAD, and MHEALTH) show that SEZ-HARN produces realistic and understandable explanations while outperforming other black ZS-HAR models in Zero-shot prediction accuracy.
Key Contributions
- Utilization of Video Data for Semantic Spaces
- Bidirectional Long-Short Term Memory (Bi-LSTM) IMU Encoder
- Human-Understandable Explanations
Lessons Learned
- Human-Understandable Explanations
- Effective IMU Signal Processing
- Model Transparency